Beyond Chess and Go: Why AI Mastering Games Could Be Good News For Everyone
BLOG: Heidelberg Laureate Forum

Lee Sedol, one of the best Go players of of all time, wasn’t expecting much of a challenge when he faced the AI AlphaGo in 2016. But despite playing excellently, he was handed a serious defeat: 4-1 to the machine. That was just the start of a true revolution in machine learning and games.
Over the past few years, we’ve seen machines learning to play myriad games (sometimes reaching a superhuman level in mere hours). From Minecraft and Shogi to Starcraft and Dota 2, machine learning is mastering games one by one – and that could be good news for us. More than just an objective in and of itself, having AI master games could function as a stepping stone towards other uses. By honing their ability in games, AIs can master skills that are important in real life, and the more skills they master, the better suited they can be for a wide array of applications.
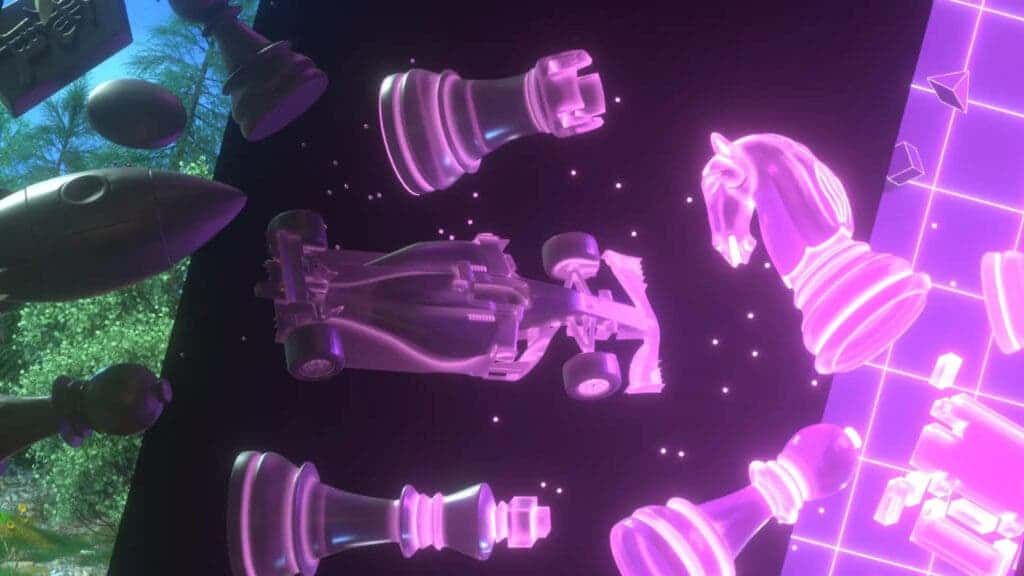
From chess to modern games – AI is ready to take on the world. Image credits: Deep Mind / press release
Conquering the unconquerable
The fact that computers are better than humans at chess is not news. Ever since Deep Blue famously defeated Garry Kasparov in 1997, the gap between computers and humans has only increased. Computer programs equipped with “traditional” algorithms (as opposed to machine learning) have outplayed humans more and more. In fact, virtually all professional chess players use computers to practice and study openings and positions.
Go is a different beast. For many years, Go was thought unconquerable by experts due to its sheer complexity (Go has an unfathomably large number of possible moves, around 10360 for a typical game, much more than the total number of atoms in the universe). Several computer scientists have approached the problem of using machines to play Go, with two researchers from the University of Missouri, Rolla, noting in 2007:
“The oriental game of Go is among the most tantalizing unconquered challenges in artificial intelligence after IBM’s DEEP BLUE beat the world Chess champion in 1997. Its high branching factor prevents the conventional tree search approach, and long-range spatiotemporal interactions make position evaluation extremely difficult.”
But while a conventional approach – like the one that Deep Blue used – couldn’t possibly work on Go (at least with today’s technology), there is another way.
Go is, in essence, a very simple game: two players with black and white pieces take turns placing pieces on intersections on a square board. But after even a few moves, the total number of possibilities gets dizzying. Image via Public Domain Pictures.
The AI AlphaGo, developed by DeepMind, uses a mixture of training obtained from both human and computer play. It employs a Monte Carlo tree search algorithm where a neural network is trained to identify the best moves by calculating the expected winning percentage of the move. But it takes things a step further. The machine learning program uses two networks: one, called the “policy network,” which decides what move will be played, and another, called the “value network,” which assesses the status of the game and who is most likely to win.
AlphaGo first learned from thousands and thousands of games played by amateur players to reach a decent level. Then, it played itself thousands of extra games, becoming stronger and improving its decision-making process game by game. Lastly, it also practiced with very strong human players to finesse its game even further. Experts in Go noted that AlphaGo played in a “conservative” style that emphasized making small, incremental gains on the board – but the machine also showed a remarkable ability to create new strategies.
In game 2 against Go champion Lee Sedol, the machine made a move that no human would have played, forcing the human player out of his chair and prompting some observers to say that maybe, just maybe, machines can be creative. Sedol echoed this by commenting after the game:
“I thought AlphaGo was based on probability calculation and that it was merely a machine. But when I saw this move, I changed my mind. Surely, AlphaGo is creative.”
Generalizing networks
Go was widely regarded as the hardest board game to master. But while mastering it was an important milestone in computer science, it was a stepping stone more than anything else. Many who followed the match were excited not just because AlphaGo won, but because the approach behind it could be generalized.
AlphaGo’s successor, AlphaGo Zero, learned how to play completely on its own.
Where AlphaGo first started by studying games from human players, AlphaGo Zero skipped this step and started out by playing against itself. At first, the games were completely random – the machine was making random moves. But it was observing what was happening and learning from it.
It quickly overtook its predecessor and within 40 days, it became arguably the best Go player in history.

The Elo rating system is a method for calculating the relative skill levels of players in many games, including Chess and Go. Credits: DeepMind / Press Release
The next iteration, AlphaZero, continued in taking things one step further. AlphaZero was only fed the rules of the game – and it wasn’t just Go. The same algorithm worked for Go, chess, and Shogi, another popular board game. It mastered chess in four hours and Shogi in two. Not only did AlphaZero beat its opponents, but according to the engineers who made it, it did so in a much more efficient way, requiring far less calculation per step than other engines.
AlphaZero also had another remarkable ability: it had style. It developed an “unconventional” and aggressive style of play, with former chess champion Garry Kasparov noting: “I can’t disguise my satisfaction that it plays with a very dynamic style, much like my own!”
As in all sports (and especially mind sports such as chess or Go), players shape their style based on the best existing players in the game, or the ones they admire most. From there on, the very best can go on to craft their own unique brand of playing, but the foundation is still shaped by other human players. AlphaZero doesn’t do that. It came with its own ideas and style.
Remember when we said chess players study positions with algorithms? Suddenly, they had a whole new range of ideas to study from. People started talking about “AlphaZero style moves” and this style of relentless, alien-like play became popular in chess.
Already, AlphaZero was a pretty general AI; but there was one more thing to drop: the rules. This is where MuZero came in.
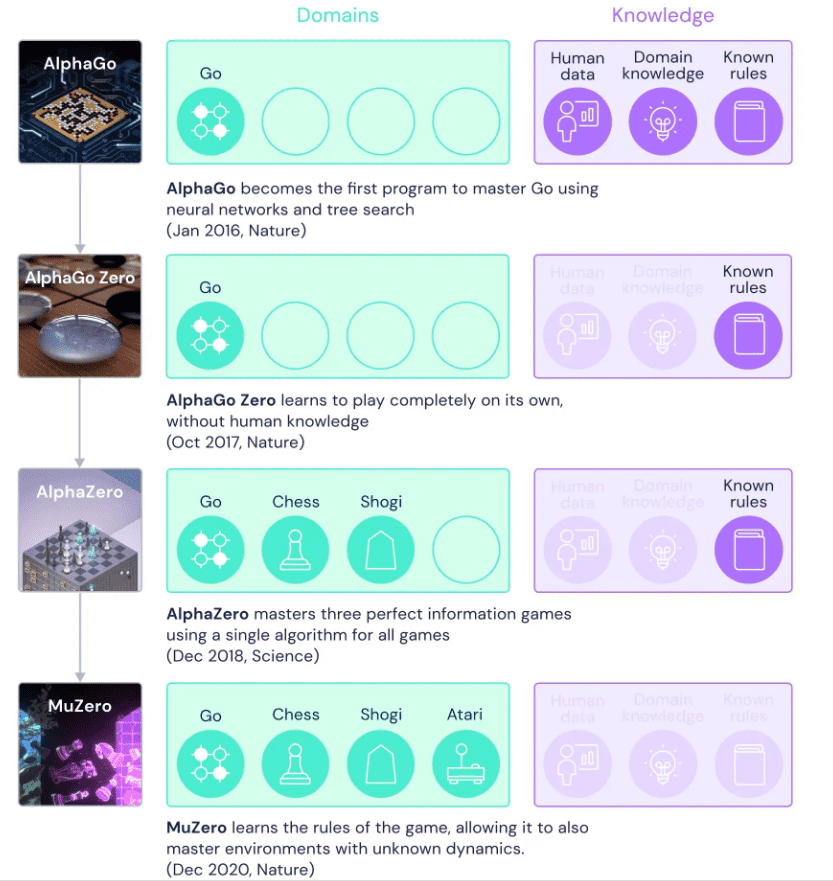
From AlphaGo to MuZero – ditching all human help in learning the game. Image credits: Deep Mind / press release.
MuZero knows nothing. When it starts out, it has no idea how to play the game, and it hasn’t studied any other games. It just tries out things and sees what happens, while aiming to win. MuZero models just three things to learn:
- The value: how good is the current position?
- The policy: which action is the best to take?
- The reward: how good was the last action?
With these three steps, MuZero was able to obtain performance similar to AlphaZero using 20% fewer computation steps per node in the search tree and was used not just in Go, chess, and Shogi, but also in Atari games. Basically, within the boundaries of a game, it builds its own model and improves on it.
Incomplete information
Go and chess may be incredibly complex games, but they have one thing that makes them more palatable to algorithms – they are “complete information” games. No matter who you are playing with, you can see the entire board and you know what is happening (if you’re competent enough). But many popular games offer incomplete information.
For instance, most strategy and role-playing computer games feature a “fog of war” element, which hides parts of the map from players when they don’t have any units around. A great example of this is Starcraft 2, one of the most popular strategy games of all time. In the game, players build various units and buildings, expand their base, and attempt to vanquish their opponents, who are trying to do the same.
AlphaStar, which as you may have guessed is the Starcraft version of the Alpha algorithms we’ve discussed previously, was able to become good enough to beat 99.8% of human players – all but the very best – even after its speed of action was capped to make it fairer. It is not clear if the project was continued after that. Another project had an AI play Dota 2, a team-based game in which two teams of five players control “heroes” that fight each other. Unlike other games, Dota 2 is collaborative, which adds an extra challenge.
Most of the algorithms playing these games are playing the games “inside” of them, directly. But some AIs have been able to figure out how to play games (and get good at it) by simply watching computer screens, something called computer vision. Basically, this means learning a game by watching someone else play it. For instance, researchers from Carnegie Mellon University trained an AI to play the classic 1993 shooter game Doom just from computer vision. Other relatively simple games, like Super Mario, Space Invaders, or Pong received the same treatment.
While still not entirely trivial, this approach has become so accessible that there are even tutorials and sample codes on how to do this online. Here’s a Youtube video showing how AI can learn how to play Super Mario by analyzing only video information.
AI has also used computer vision to tackle more complex games. An algorithm from OpenAI looked at 70,000 hours of Youtube videos of the survival crafting game Minecraft. Within Minecraft, the machine was able to cut down trees to collect logs, craft the logs into planks, and then craft the planks into a table. It was also able to create more complex tools like a diamond pickaxe and deploy various tricks such as “pillar jumping” (a common behavior in Minecraft of when you elevate your self by repeatedly jumping and placing a block underneath yourself).
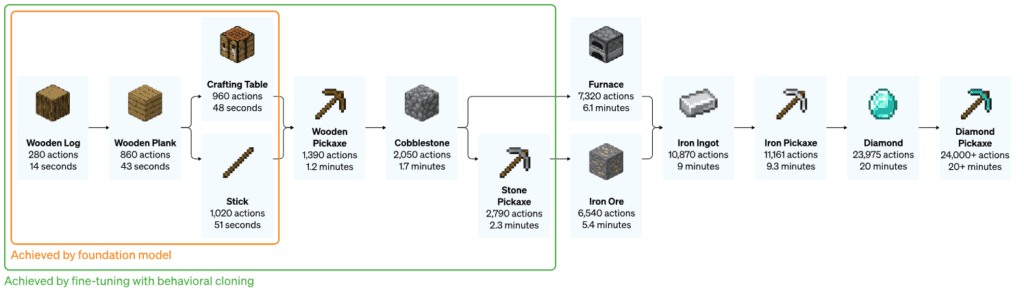
It takes a proficient player over 20 minutes of fairly complex sub-tasks to create a diamond pickaxe in Minecraft. Image credits: OpenAI.
Beyond the game
At first glance, this may seem inconsequential. Sure, it’s cool that we can make algorithms that can play games; you could argue that there is value in that alone, or in algorithms helping people improve their own performance at these games – but aside from that?
Computer scientists rarely regard mastering games as an objective in itself, they see it more like a practical exercise or a stepping stone towards more practical applications. For instance, the algorithm that played Dota 2 was used to power a human-like robot hand with a neural network built to manipulate physical objects. AlphaZero has already been applied to a range of complex problems in chemistry and quantum physics. As a general algorithm, it can be deployed to a number of different fields, including the field of protein folding.
Bioscientists have tackled the complex problem of protein folding for decades. A protein’s shape decides the protein’s function, but it’s hard to predict. AlphaFold, using algorithms similar to those deployed for chess or Go, was first released in 2020, predicting the structure of 98% of all human proteins. The ability to accurately predict protein structures would be a massive boon for biological and medical research, paving the way for quicker and more advanced drug discovery, as well as a better understanding of the building blocks of life. Now, this database of proteins has been expanded to include the proteins in almost all other organisms on Earth, including animals, fungi, plants, and bacteria. That advancement alone is probably enough to justify all the work, but Alpha and other game-playing AIs are just getting started in the real world.
We have machine learning algorithms that are able to become proficient at various tasks without even knowing what they are tasked with and algorithms that are able to learn tasks just by watching others. Sure, training these algorithms still takes a lot of time and energy; it’s not perfect, and it’s pretty inefficient, but more and more efficient versions are constantly being developed.
Artificial Intelligence is still somewhat of a misnomer; it’s not exactly intelligence in the way you or I have intelligence. AIs are good at performing singular or limited tasks in a specific environment, but as they get better at more complex and realistic games, they are also getting ready to step outside of a controlled environment and into the real world – or at least, some corners of the real world.
But perhaps there is another, more human lesson that can be drawn from game-playing AIs, one that’s less about algorithms and more about humanity.
The Lee Sedol vs AlphaGo was a watershed moment for machine learning in games in more ways than one. It took the human champion out of his comfort zone. Sedol would go on to lose the match and three years later, having accomplished everything he could in the game of Go but realizing he cannot beat the machines anymore, he withdrew from Go. “Even if I become the number one, there is an entity that cannot be defeated,” he would go on to say. We could leave it at that: a bitter ending for humans competing in the world’s oldest and most complex game. But there is more to the story. Sedol played a fantastic match. In the one game he did manage to win, he played a genius move that took everyone by surprise, including Alpha Go. Would he have found it if he had not been playing this AI? Maybe, maybe not.
Here’s the thing: the AI may be capable of superhuman performance; it may make genius moves – but humans can, too. AI is rarely (if ever) about bettering or replacing humans, it is about helping humans reach their potential, and maybe even surpass it. We may soon never again be able to beat machines at games, any games, regardless of how complex they are, and that’s fine. Humans are flawed, but so are AIs. They can’t pass the Turing test, understand reality, or make fun of one another. Humans are generalists, AIs are specialists, and we all have our own limitations.
AI is great at optimizing narrow strategies and finding patterns in existing data. Humans are better at conceptualizing and dealing with abstract matters. Together, we can accomplish great things. Complementing human ability – not surpassing or replacing it – is the goal.
Quote:
Yes, but the weak abstraction/generalization capabilities of current AI systems are worrying in important areas. For example, reinforcement learning is conceptually very attractive, as it does not require a model of the area of application, all it needs is enough samples. But the sample inefficiency (instead of 10 samples, it takes thousands of samples) also means that reinforcement learning cannot be used to train robots directly, as a robot becomes defective when it tries all kinds of movements for hundreds of training hours. Due to this, a simulated robot has to be trained today by reinforcement learning and below the simulation is transferred to a real robot. The problem here is that the transfer itself is difficult, because the simulation always misses some limitations of a real robot.
My prediction: Future AI systems must and will be more sample-efficient, as training cannot access big data in many important applications.
Supplement: Current Reinforcement Learning is not sample efficient, but this could change in the near future as the arxiv-article A Walk in the Park: Learning to Walk in 20 Minutes With Model-Free Reinforcement Learning demonstrates, where we find the following abstract:
Conclusion: My prediction that future AI systems will be more sample-efficient is already flourishing (prematurely), because even if you use all the refinements of reinforcement learning described in countless articles, published recently, you get much more sample-efficient learning systems than just a few years ago.
Es ist ein wenig betrüblich, wenn sich Spiele wie Schach und Go als minderkomplex erweisen, dem menschlichen Geist widersprechend, Dr. W war zumindest manchmal gut in einem dieser Spiele.
Es liegen jeweils Spiele mit sog. vollständiger Information vor.
Auch Backgammon ist sozusagen erledigt.
Ansonsten gibt es noch Spiele wie Poker oder NetHack, Poker ist in einigen Varianten sozusagen ausgerechnet, NetHack wird gerade so bearbeitet. als sozusagen König aller Spiele, auch Terry Pratchett ist auf dieses Spiel aufmerksam geworden :
Es gibt auch gewisse Bemühung in puncto AI hier schlau zu werden, ein wesentliches Problem besteht für den Spieler bei NetHack darin, dass er jederzeit sozusagen alles machen kann.
Eine Turn- oder Zugbasiertheit liegt ähnlich wie im Schach vor, doch kann in jedem Turn sozusagen unendlich viel gemacht werden.
Mit freundlichen Grüßen
Dr. Webbaer
PS zu :
Derartige Mehrschichtigkeit ist bundesdeutsch vor etwa vier (drei?) Dekaden bereits von Ingo Althöfer gedacht worden.
Sein sog. Drei-Hirn-Konzept meinend.
Ingo springt bundesdeutsch und medial beachtet auch heute noch herum.
(So schaut’s besser aus, Dr. W hat (wieder mal) an falscher Stelle ein Kleiner-Zeichen für die Zwecke der Textauszeichnung gesetzt.)
Artificial General Intelligence (AGI) versus narrow AI with superhuman abilities
AlphaGo, Alpha Zero and MuZero all have superhuman abilities in a narrow area, namely in perfect information games such as chess and go. The use of Monte Carlo Tree Search in combination with reinforcement learning seems to be the best approach to play games and to solve sequential decision-making problems.
Forecast: Even future AGI systems will not replace the neural Monte Carlo tree search, as general intelligence is not only more general, but also weaker than specialized programs for special applications. The same applies to AlphaFold, a program that has already decoded the 3D structure of most human proteins. AlphaFold will prevail through more general problem solvers.
Outlook: Systems with human intelligence are far in the future, but when they emerge, they will not replace specialized systems such as AlphaFold or MuZero. Instead, an AGI system decides like a human being and knows when it should use specialized programs such as AlphaFold to solve a problem.
@ Kommentatorenfreund “Martin Holzherr” (die doppelten Anführungszeichen nur deshalb, weil Sie ein als solches unerkennbares Pseudonym verwenden) und hierzu kurz :
Bei den beiden genannten Programmen ist es so, dass AI eingesetzt worden ist, der Mensch oder allgemeiner formuliert : das erkennende Subjekt (mit bes. Fachwissen), sozusagen wie ein Jockey (“Johannes”) auf ihnen gesessen hat, um das Programm in eine günstige, besonderer Gewinnung von Erkenntnis dienende Richtung zu scheuchen sozusagen, damit es sich nicht verrennt (AI kann sich verrennen).
Mit Gewinn.
Würde “Artificial General Intelligence (AGI)”, als Großprodukt sozusagen, als Leben sozusagen, so auftauchen können, hätte es aus diesseitiger Sicht in der Tat Spezialprogramme wie zitiert zu nutzen, sozusagen.
SCNR
Dass hier irgendwie Evolution informatorisch nachgebaut wird, könnte klar sein.
Randbemerkung :
Dr. Webbaer mag das :
-> https://scilogs.spektrum.de/gehirn-und-ki/
und den hier :
-> https://scilogs.spektrum.de/gehirn-und-ki/author/konecny/
(Gerne mal reinhören, reinlesen, es werden auch audiovisuelle Dokumente bereit gestellt, ga-anz bes. Zustimmung des Schreibers dieser Zeilen ist nicht gemeint.)
Mit freundlichen Grüßen
Dr. Webbaer
@Dr. Webbaer (Zitat):
Dass bei AlphaFold und Muzero AI eingesetzt wurde, bedeutet konkret, dass Deep Learning eingesetzt wurde. Deep Learning ist eine Methode um aufgrund von komplexen, miteinander vernetzten Eingabeparametern eine Entscheidung zu treffen. Deep Learning Systeme sind dann, wenn die Eingabedaten die „richtigen“ sind, darin, die richtigen Entscheidungen zu treffen, ebenso gut oder besser als Menschen. Doch wie erhält Muzero die „richtigen“ Eingabedaten? Durch Verwendung von Monte Carlo Tree Search, also die Verwendung eines Spielbaumes, der besonders gut geeignet ist um von Deep Learning ausgewertet werden zu können. Ohne einen solchen Spielbaum, also ohne Monte Carlo Tree Search würde Deep Learning nicht genügen um erfolgreich Schach oder Go spielen zu können.
Mit andern Worten: AI anzuwenden bedeutet heute vor allem Deep Learning anzuwenden. Doch AlphaFold oder Muzero bauen auf mehr auf als auf Deep Learning. Sie verwenden Methoden um Deep Learning die „richtigen“ Daten zu liefern, die dann die Basis von Entscheidungen sind.
Im übrigen lese ich, überfliege ich, jeden Tag die arxiv-Artikel zum Thema Artificial Intelligence, halte mich informiert, was die Firma DeepMind neues zu bieten hat und lese auch Artikel von Konecny und anderen hier auf scilogs.
Der hier ist aus diesseitiger Sicht schon vglw. cool :
Dr. W hat wie folgt vorgebaut : ‘ga-anz bes. Zustimmung des Schreibers dieser Zeilen ist nicht gemeint’
Dr. W mag seinen Inhalt und Sound sozusagen, eitel :
-> https://scilogs.spektrum.de/gehirn-und-ki/author/konecny/ (Wer so-o sprechen kann, muss doch etwas zu sagen haben, oder, ODER, ODER?
(Und eitel.)
—
Spaß beiseite, Dr. W mag so, Ihre Nachricht :
“Monte Carlo” meint ja eine zugebaute Zufälligkeit.
Nicht mehr.
Es geht hier um die zielgerichtete, an Interessen gebundene Teilnahme von letztlich um Erfolg bemühten Subjekten, die sich sozusagen nicht anders zu behelfen wissen, statt eigener Verständigkeit sozusagen robotische Rechenfähigkeit hinzuzubauen, gesondert anzufragen, um sich beraten zu lassen.
Mit gutem Erfolg teilweise, der Beratende bleibt erst einmal ein Rechner, der gerne auch im sozusagen EVolutionären flexibel bleiben darf, i.p. Rechnung und Bewertung dieser Rechnung.
Vorgegeben von Anderen.
Die ‘Daten’ sind bekannt, es geht hier sozusagen um geordnete Variabilität bei ihrer Bewertung, was se-ehr schwierig ist und gerne von sog. AI unterstützt werden darf, weil ganz besonders rechnend, sozusagen, als Tool. [1]
Mit freundlichen Grüßen
Dr. Webbaer
[1]
Der mit dem hier nicht aneinander geraten möchte.
Vielleicht später.
PS :
Dr. W weiß jetzt nicht so genau, ob Alles korrekt textlich ausgezeichnet war, hat sich abär bemüht.
Wobei es schon so ist, dass Vokale gar achtfach ausgesprochen werden können, das ‘A’ bspw. chinesisch, wie sich Dr. W von einer Mitarbeiterin vorführen ließ.
JFYI.
@Dr.Webbaer (Zitat):“ “Monte Carlo” meint ja eine zugebaute Zufälligkeit.„
Ja, der Spielbaum wird traversiert, wobei der Abstieg in einen der Unteräste des Baums mit einer gewissen (gerechten) Zufälligkeit erfolgt. Gemäss Wikipedia gilt für Monte Carlo Tree Search :
MCTS ist also geeignet, wenn eine Folge von Entscheidungen getroffen werden muss und jedesmal eine Auswahl unter n Möglichkeiten besteht. Das entspricht einem Entscheidungs- oder Spielbaum.
Video games are awesome. The small part of my brain that still has game controls on my phone knows this fact and is screaming it in the back of my head. Now you get Casino Softwarehersteller and learn more new casino games. Because even though it feels like we’re doing exactly that, more often than not, most people are playing it safe with their game design decisions. And I don’t mean that in a derogatory way.
thank for sharing