Hugo Duminil-Copin: The Maths, the Man
BLOG: Heidelberg Laureate Forum

Hugo Duminil-Copin will be a name familiar to mathematicians worldwide. He was awarded the 2022 Fields Medal for his work in statistical physics, making him just about as famous as a mathematician could get. Meeting him, though, you would never know it. He is warm and down-to-earth, nothing like the stereotypical picture of a genius that one might expect him to project.
This year was Duminil-Copin’s first time at the Heidelberg Laureate Forum, and he was very generous with his time, appearing as part of a panel discussion on the Thursday, giving a lecture on the Friday, and still finding time to talk to the young researchers and give a press conference. Throughout the week, I got to learn a lot more about Duminil-Copin, and was able to discover a bit more about the maths behind the man and the man behind the maths.
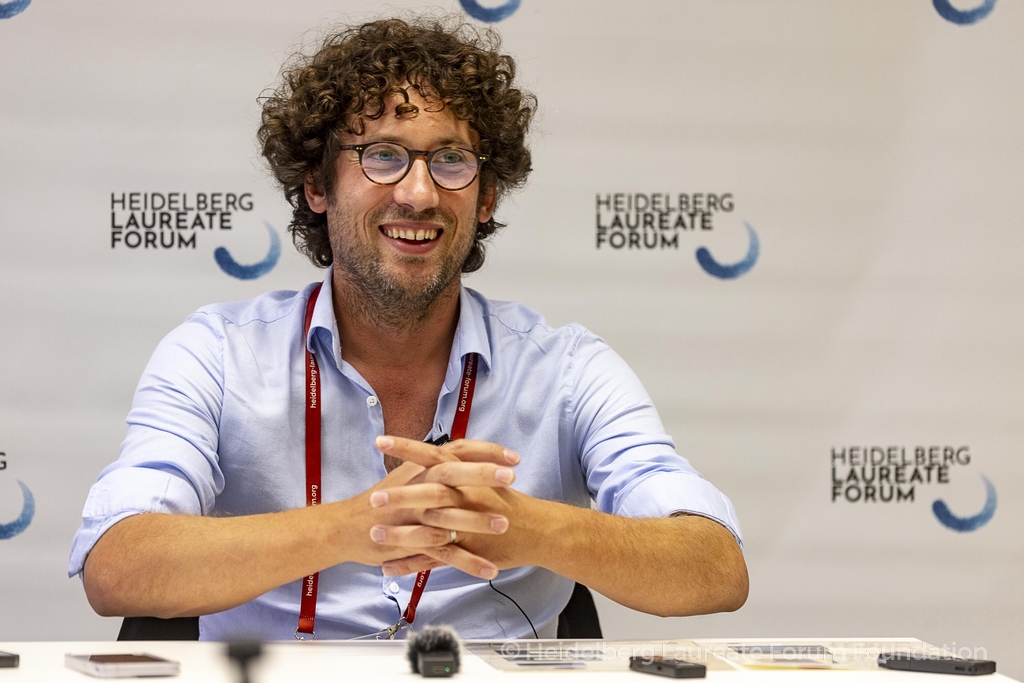
The Maths
Duminil-Copin works in statistical physics, looking at the probabilistic theory of phase transitions. This is a bit of a mouthful but what it essentially means is that he uses statistical models to investigate changes in state. The work which won him the Fields Medal focussed on a specific mathematical model: the Ising Model.
The Ising Model, as Duminil-Copin explained in his lecture, looks at alloys composed of precisely two elements (binary alloys) and attempts to explain their magnetism. Magnets are made of dipoles, which have a magnetic moment known as “spin”. Spins can point in one of two opposing directions. When arranged in a lattice, each spin will interact with its neighbours. When neighbouring atoms spin in the same direction, they have a lower energy and hence the system would naturally tend to this state. The introduction of heat, however, can disrupt this, which can lead to a variety of structural phases and hence there could be a phase transition.
The Ising model is named after Ernst Ising, who was set the problem by his supervisor Wilhelm Lenz. Ising proved in 1925 that there was no transition between phases in the one-dimensional case. It took until 1944 for Lars Onsager, an American physical chemist and recipient of the 1968 Nobel Prize in Chemistry, to provide a solution for the two-dimensional case. The amount of time that it took between Ising and Onsager’s solutions gives a useful insight into the comparative difficulties of the two cases. It is not surprising that third and fourth dimensions proved to be harder still. In fact, the solutions in three and four dimensions eluded mathematicians, until finally Duminil-Copin was able to compute the continuity and sharpness of the phase transition in three dimensions. He has made great strides in the fourth dimension as well, which is also mentioned in his Fields Medal citation.
The Models
Much of Duminil-Copin’s work deals with probability theory, and he pointed out in his press conference that probability is something people use in their daily lives, even without realising it. For example, you may assess the probability that it might rain, when deciding whether to wear a coat in the morning. Or you might work out the chance that your bus will get caught at a red light when telling a friend how long your journey will take.
Thinking probabilistically is second nature to people. Duminil-Copin elaborated that in order to work with complex information, our brains need to be able to assess uncertainty. “The irony is that we’re catastrophically bad machines of consciously understanding probability,” he laughed. When it comes to independence, conditionality, and assessing small probability events, humans slip up frequently. One only needs to look at the birthday paradox to see this. The birthday paradox is the counterintuitive fact that in a room of 23 or more people, there is a greater than even chance that at least two share a birthday. Probabilistically we can show that this is true, but to many people it just feels wrong.
The way the human brain deals with probabilities and decision making closely resembles a technique mathematicians regularly use – it simplifies. When determining the trustworthiness of a new friend, there is a wealth of information that could be drawn upon – the thousands of clues in their body language, whether you have mutual friends, the veracity of what they are saying. It is humanly impossible to think through every factor, and even if it were possible, it would take a long time. So, our brains filter the data, sacrificing some accuracy for speed.
We do the same in mathematics, and this is called creating a mathematical model. Mathematical models attempt to describe a situation in the language of maths. This will normally involve some sort of equation, but it does not have to. Models are almost always a simplification of reality but can still give a very useful insight into what is going on. An example of a model which shot up in popularity in 2020 is the SIR model. This model explains the dynamics of diseases – such as COVID – with the S representing the susceptible population, I representing the Infected population, and R representing the Removed population, i.e. those who are no longer able to catch COVID, hopefully through immunity but sometimes through death.
Duminil-Copin explained in his lecture the key ingredients needed to create a mathematical model, such as the Ising Model: First, you need to start with an experience, something you notice. Then you want to define your parameters. Duminil-Copin had a catchy slogan for this stage: “Be wise, discretise.” Finally, you introduce a bit of randomness into your model, take limits (see what happens when you get closer to the extreme cases of the model) , and try to break the symmetry.
The Methods
In the Ising model, the spins are arranged in a lattice and can each take one of two values, \(+\) or \(-\). Therefore, if there are \(L\) spin sights, there are \(2^L\) possible states that the lattice can be in. This lends the problem to a simulation using Monte Carlo methods.
Monte Carlo methods are computer algorithms that repeatedly run randomised simulations to get a sense if what results can be expected. Often an average will be taken over the many tests that are run. By running the experiments as a computer simulation, time and money are saved. This allows orders of magnitude more results to be obtained than if the experiments were done physically. The law of large numbers gives that the distribution of the repeated events should tend to the true distribution of the random variable.
In the case of the Ising model, the simulation runs as follows. The experiment starts in a (possibly randomly chosen) state. Then a random spin site \(v\) is selected according to some probabilities \(g(\mu,v) = \mathbb{P}(v \text{ is chosen }|\text{ in state } \mu)\). Normally it is assumed that every spin site has the same chance of being chosen: \(g(\mu,v) = 1/L\) for all \(v\).
Now this is where it gets a bit fiddly. Let us call the state with spin site \(v\) flipped (from \(+\) to \(–\) or from \(–\) to \(+\)) \(\nu\). We define some acceptance probabilities \(A(\mu,\nu) = \mathbb{P}(\text{ state }\nu\text{ is accepted given the previous state was state }\mu)\). Then we randomly decide whether to move to state \(\nu\) or stay in state \(\mu\) with probability \(A(\mu,\nu)\) that we swap to \(\nu\).
We repeat the process until a defined end point. Normally in the case of the Ising model, this end point is when all the spins are aligned, i.e. the alloy has become ferrous (magnetic).
The meat of this method is in determining the probabilities \(A(\mu,\nu)\). These probabilities rely on a parameter \(\beta\), given by \(\beta = \frac{1}{k_B T}\) for \(T\) the temperature and \(k_B\) the Boltzmann constant. Therefore, \(\beta\) is the reciprocal of the “thermodynamic temperature”. The other parameters which \(A(\mu,\nu)\) depend upon are the energies at states \(\mu\) and \(\nu\), denoted \(H_\mu\) and \(H_\nu\) respectively. Through a principle known as “detailed balance” we get that
\[A(\mu,\nu) = \begin{cases} e^{-\beta(H_\nu – H_\mu)} & \text{ if } H_\nu – H_\mu >0, \\ 1 & \text{otherwise.} \end{cases} \]
I will not go into the intricacies of what detailed balance means, but we can see that the greater the increase in energy, the less likely it is that we move to the new state. On the flipside, if the new state has less energy than the initial state, we always swap to it. This fits with our intuition, as nature tends to move towards configurations with minimal energy.
Another fact that can be seen from the expression for the transition probabilities A is that the as the temperature increases, it becomes more likely that we keep the new state, even when the new state is of higher energy. Again, this makes sense because a higher temperature means that there is more energy in the system.
There is a particular name in mathematics for a series of states with transition probabilities between them. What I have described above is known as a Markov Chain. In a Markov Chain, as seen above, the probability of transitioning to a future state can depend only on the current state. Knowing this means that the known mathematical properties of Markov Chains can be used to analyse the Ising model, giving more tools in a mathematician’s arsenal.
Unfortunately, as with any model, this is a massive oversimplification, and it is not sufficient in order to determine phase transitions in three or four dimensions. To do this, Hugo Duminil-Copin had to employ tools from quantum field theory among other disciplines, in the process solving conjectures that had been open since the seventies – years before he was born in 1985.
The Man
Duminil-Copin comes from a family of teachers and athletes. His mother was a dancer who then became a primary school teacher, and his father was a sports teacher. He has inherited his parents’ love for sport, and famously his childhood dream was to become a handball player. In fact, it was only with one year remaining at high school that he decided instead to become a mathematician.
According to Duminil-Copin, one learns sport in the same ways as one learns maths – by repeating the same movements again and again. Duminil-Copin also explained how sport helped him become adaptable and gain resilience. He swapped sports every two years or so, which helped him become very good at starting from scratch and learning something new.
Duminil-Copin derives the same enjoyment from maths and sport. “You get pride in maths because you sweat,” he explained. He also is a fan of the collaborative aspects both have the capacity for: “I mostly like team sports. I think that math is a team science.” Duminil-Copin stresses the importance of his collaborators, noting that none of the papers for which he earned his Fields Medal were written alone.
Hugo Duminil-Copin is not afraid to show his fallibility and his humanity. When asked by a journalist about how it felt to become a professor at such a young age, he was keen to point out that up until that point, he had done everything at the standard age. He explained how he thinks it is incredibly unhelpful to only talk about those who are successful from a young age and have illustrious careers from the moment they begin. He strongly believes we need to break the fantasy that mathematicians understand everything immediately; he tells without a trace of embarrassment of how he came second to last in a maths test in his penultimate year of high school.
Hugo Duminil-Copin breaks many of the stereotypes of mathematicians. He is sporty, he is down-to-earth, he is young and charismatic. So where does that leave his view of himself as a mathematician? In this respect, Duminil-Copin breaks one more misconception about maths, namely that it is not a creative subject. “I like to think of myself as an artist more than as a scientist discovering things,” he says. So, there it is from the lion’s mouth. Hugo Duminil-Copin: the very model of a modern mathematician.
Es geht darum, wissenschaftlich und der Idee der Falsifikation folgend, eine Hypothese, eine sog. Nullhypothese, die Hypothese meint die Unterstellung, die Möglichkeit, sie darf bis muss rational (vs. irrational) gehalten sein, so-o mit den Mitteln der Empirie, mit Experimenten, die Daten, Gemessenes und das Weltliche meinen, naturwissenschaftlich soz. so-o zu beschießen, dass die Komplementärhypothese wahrscheinlicher wird, wichtig ist hier die Gegensätzlichkeit zur zu testenden Hypothese
.Zu :
Ganz genau.
Mit freundlichen Grüßen
Dr. Webbaer
Bonuskommentar, sich diesen gerne übersetzen lassen, danke, hierzu :
Einfachheit ‘opfert’ sich sozusagen der ‘Einfachheit’, nicht der ‘Geschwindigkeit’. [1] [2]
.
Einfachheit ist ein im wissenschaftlichen Sinne anzustrebendes Konstrukt.
Sicherlich ist zum Wesen der menschlichen Verständigkeit auch so anzumerken :
-> Das Volumen eines menschlichen Gehirns liegt bei einem Mann bei durchschnittlich etwa 1,27 Litern, bei einer Frau bei etwa 1,13 Litern. [Quelle].
MFG – WB
[1]
-> https://de.wikipedia.org/wiki/Sparsamkeitsprinzip
[2]
Die Moderne Naturwissenschaftlichkeit nähert sich der Welt, im oben genannten skeptizistischen Sinne, an Beleg-Lagen gebunden, auch näherungsweise, ausschnittsartig und an Interessen (!) gebunden.
Wissenschaftlichkeit ist eine Veranstaltung.
heute möchte ich meine Begeisterung für Perlennation mit Ihnen teilen, einen Online-Shop, der zeitlosen Echtschmuck aus hochwertigen Naturperlen anbietet. Perlennation legt großen Wert auf verantwortungsvolle Herstellung und Nachhaltigkeit und ihre Schmuckstücke sind ein wahrer Ausdruck zeitloser Eleganz.
Besuchen Sie die Internetseite perlennation.de und lassen Sie sich von der beeindruckenden Kollektion inspirieren. Es lohnt sich!
Bis dann,